words Al Woods
Quality is what we want and aim for in everything we do. And there certainly is no exception when it comes to something as important as data. The growing use of various data for business proceedings means that ensuring data quality is as paramount as anything else we do in business.
Taking a quick look at how excellent quality of data is achieved and used will allow us to better understand the growing importance of data in business today.
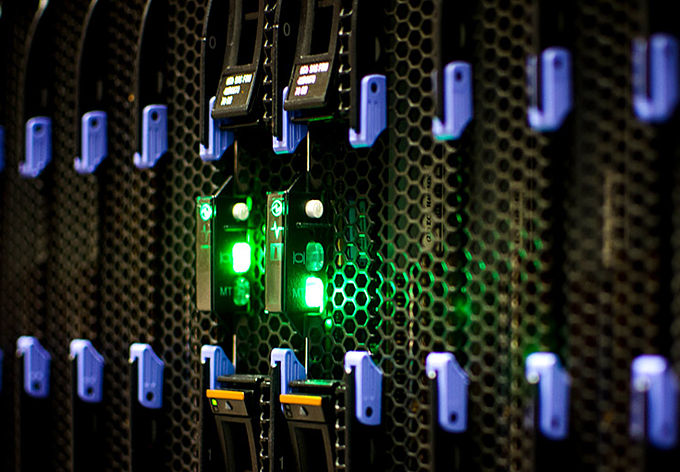
Ensuring data quality: an ongoing process
Making sure that your company datasets are of the highest quality that can reasonably be expected requires constant catering. The process of nourishing data to improve its quality usually involves multiple steps. However, each step will be more efficient if we are dealing with a considerable level of data quality, to begin with.
It means that a lot of data handling processes that eventually lead to improved databases work better with data of higher quality. This idea is better illustrated by the following 4 use cases for data quality.
1) Data standardization. As data is collected for different purposes, from various sources, and using different methods, it is often stored in different formats. Standardization is the process of organizing differently recorded data into consistent structure throughout the database. This makes the data more easily accessible and ready for use, as well as allows to avoid errors arising from inconsistencies. However, data standardization works better when information in the datasets is already recorded conforming to standards of data quality. That is, the fewer data fields are left empty, the fewer typing errors there are, the more smoothly and productively will the standardization process go.
2) Data cleansing. Here is where data cleansing comes in. To make sure that the data quality is of high enough level before standardization, it can be cleaned to remove some major flaws. Cleansing consists of detecting and correcting the incorrect data records. Where correction is not possible, the records would be removed so as not to further corrupt the dataset. Different models of data cleansing may be employed, depending on what we wish the final result to look like. For example, we may want to remove all incomplete records or leave them if there are no errors in the fields that are filled in. When we want data to be corrected, it is more likely to get the right results when high-quality data is available in some of our datasets which can serve as a reference point for validating other records.
3) Data profiling. This is the process of examining data in a particular dataset to draw its profile, that is determine what is the structure, types, and relationships of the data. This is done for various reasons, including quality assessment and classification, to make particular data units easier to find. It also helps in determining whether the data is compatible with information recorded elsewhere and can be used for different purposes. On the one hand, a data profile is used to discover the existing data quality. On the other hand, the better is the quality, the more can be achieved by data profiling as it would immediately result in making the data more accessible and finding new ways to utilize it.
4) Data governance. This term might often be encountered as referring to the governmental and international regulations and guidance of data storing and using. On the company level, it refers to data management rules ensuring data quality on all levels of usage. When the data of the enterprise already conforms to the basic standards of data quality, data governance is made much more efficient. Furthermore, a lot of resources is saved for utilizing data to create value when they are not required for fixing various problems created by low-quality data, including security threats that it poses.
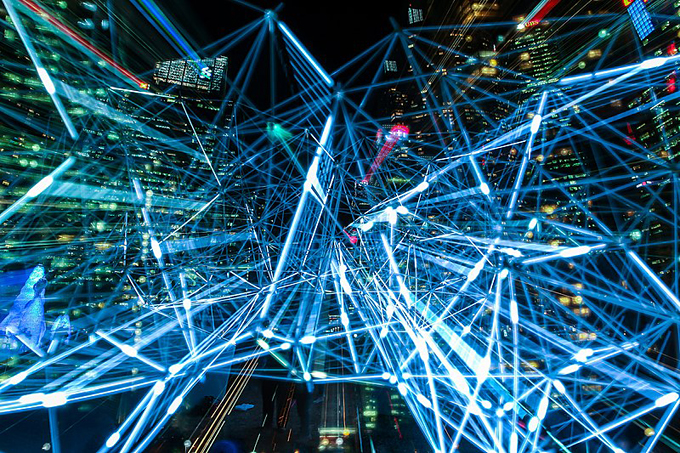
The meaning of quality
The above use cases show how data perpetually goes through circles of improvement to achieve and retain high quality. These processes are employed to make sure that the information stored in your datasets is of the highest quality and usability. The list above also hints at what it means for data to be of high or low quality.
Firstly, high-quality datasets are mostly free from inaccurate, corrupt and unreadable information. Secondly, the data stored in the database is not repetitive and easy to access. Finally, the data is to utilize for the purposes of the business either alone or in combination with data from other sources.
Naturally, this explanation of data quality is not nearly exhaustive. What primarily should be added to it depends on the kind of business and the type of data in question.
Quality has no boundaries
As a concluding remark, it should be noted that it is highly unlikely to achieve perfect quality data. Something can always be improved. Therefore, the quest for high-quality data is a non-stopping process that requires a lot of attention but also brings enormous value to those that pay it.